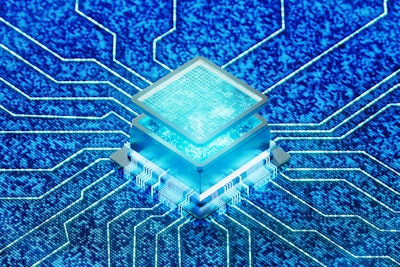
Artificial Intelligence (AI) has transformed the Original Equipment Manufacturers (OEM) sector from a futuristic concept into a competitive necessity. With 96% of manufacturers planning to increase AI investments by 2030, it's crucial to understand both AI's evolution and current capabilities in manufacturing.
The Early Days: Expert Systems and Automation
AI's historical journey in manufacturing began during World War II with Ford's use of electro-mechanical vision systems to inspect components, significantly reducing production cycle times. The post-war era saw further advancements with robotics and complex AI-driven processes like Failure Modes and Effects Analysis (FMEA) and Statistical Process Control (SPC), becoming integral to manufacturing.
The Rise of Machine Learning and Advanced AI
In the 1990s, machine learning algorithms began to replace rule-based systems, introducing adaptive learning capabilities for more complex problem-solving and optimization in manufacturing processes. In packaging, AI has been instrumental for decades, revolutionizing processes from sorting work to traceable labeling. AI-driven validation systems, automated line inspection platforms, and depalletizing systems have greatly enhanced efficiency and traceability, ensuring product safety and regulation compliance.
The Current Era: IoT and Smart Manufacturing
The integration of the Internet-of-things (IoT) and advances in robotics have led to pervasive digitalization, ushering in the era of Smart Manufacturing and Industry 4.0. In this period, AI has played a vital role in enhancing throughput, quality, and cost efficiency while ensuring a safe working environment. The implementation of AI, particularly machine learning, has been critical in processing the vast amounts of data generated in manufacturing, leading to significant improvements in productivity, quality, flexibility, safety, and cost.
- AI-Enhanced Manufacturing and Packaging Efficiency: AI plays a pivotal role in manufacturing and packaging by optimizing the performance of machinery through advanced analytics and machine learning. This includes predictive maintenance, which anticipates equipment failures before they occur, and process optimization, where AI algorithms continuously analyze production data to fine-tune operations. In packaging, AI-driven systems can dynamically adjust packaging processes based on real-time demand and supply data, ensuring more efficient resource utilization and reducing waste.
- AI-Driven Interactive Packaging: In the realm of smart packaging, AI takes consumer engagement to the next level. Beyond just being interactive, AI-enabled packaging can personalize consumer experiences. For instance, AI can analyze consumer interaction data gathered through QR codes or augmented reality features to offer tailored content, such as personalized instructions, product recommendations, or augmented reality experiences. This not only enhances the customer experience but also provides valuable consumer insights to manufacturers.
Generative AI and Process Manufacturing
In process manufacturing, Generative AI is emerging as a technology with the potential to significantly transform the industry. It is recognized for its advanced data interpretation and content creation abilities, offering new approaches to operational strategy refinement. The capabilities of Generative AI in product design and development are particularly promising. By employing machine learning algorithms, it could potentially explore a vast array of design solutions, aiming to enhance operational efficiency, structural integrity, and optimize material usage.
The application of Generative AI in process manufacturing holds the promise of surpassing traditional design limitations, facilitating innovative and unconventional designs. With the ability to process specific design goals and constraints, it could generate diverse solutions that align with these parameters. This process, if realized to its full potential, could lead to a significant increase in design efficiency, open new avenues for innovation, improve overall performance, and potentially result in considerable cost savings. While still in the nascent stages, the capabilities of Generative AI suggest a transformative future for process manufacturing, where efficiency and innovation could redefine industry standards.
Current Limitations of AI in Manufacturing
While AI has brought numerous advantages, it also has limitations:
- Cost: The initial implementation of AI can be expensive, particularly for startups and small companies. Ongoing expenses include maintenance costs and cybersecurity measures.
- Expertise Requirement: AI requires skilled experts for sophisticated programming and maintenance. With AI experts in high demand, finding and employing such professionals can be challenging and costly.
- Security Vulnerabilities: As AI systems become more sophisticated, they also become more vulnerable to cyberattacks. A breach can disrupt production lines and, in severe cases, shut down a business. Continuous updates and investments in security measures are essential to protect against these threats.
The evolution of AI in packaging and process manufacturing signifies continuous innovation and adaptation. While AI has revolutionized many aspects of these industries, understanding its current limitations is crucial for realistic and strategic implementation. Future articles will delve deeper into practical applications and upcoming AI trends in these specific sectors, providing comprehensive insight into this transformative technology.
David McGraw is a Senior Director at Alvarez & Marsal Private Equity Performance Improvement Group LLC.